What developments in AI mean for Language Education

by Michael Cai on Aug 7th, 2023
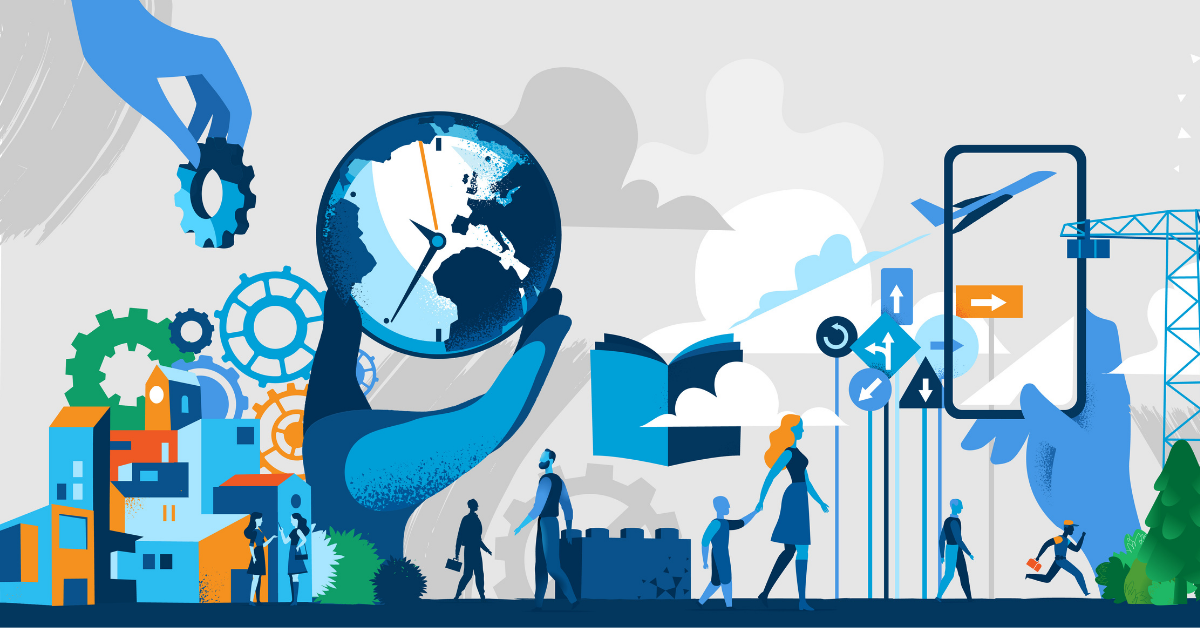
Introduction
The 21st century has seen a recent boom in AI-related products and discoveries. From new transformer-centered model architectures to Dall-E to ChatGPT, new innovations are now rapidly expanding the horizon of what is possible for artificial intelligence and its applications. Recently, natural language processing (commonly referred to as NLP) has been in the forefront of this so-called “AI revolution,” and for good reason too. NLP products and services (ChatGPT and other generative AI chatbots, for instance) have brought to many people’s attention the practical, everyday uses AI can have. Moreover, creations centered around NLP have fundamentally altered — and will more likely than not continue to alter — many fields, particularly the field of education.
Although almost every educational subject would benefit a great amount from these innovations in NLP, this article will focus on applications in world language education. This is because NLP and current generative AI technologies pair very well with themes present in foreign language learning. The process of learning a language strikes a good balance between black-and-white, almost “binary” logic and fluid writing which offers more leeway: firstly, a main aspect of picking up a new language is applying logical rules (such as grammatical laws) that have a relatively well-defined set of correct and incorrect answers. Indeed, this aspect is similar to qualities present in math, science, and other STEM subjects. That said, the language classroom rarely explores the nitty gritty of logical relations present within these fields, ones that can be quite complex and may result in glaring issues for an AI model not trained to solve a specific problem. Language learning offers leeway in this sense because within the context of fluid writing, logical errors (which are bound to happen for any complex and nuanced subject) would be less detrimental.
Interactive Learning
Though AI technology has definitely impacted language learning, technologies like Quizlet or Duolingo have made large ripples in the field prior to any mass-scale wave of AI innovation. So, then, what does the future look like?
Again, these technologies have already made large improvements through promoting interactive learning. However, standard, rule-based algorithms can only go so far when it comes to simulating a sense of interaction. AI, on the other hand, could exponentially augment language learning in this respect. One reason is the nonlinearity of most state-of-the-art AI models. Unlike a typical algorithm, which applies a very well-defined set of instructions on an input to obtain an output, AI models oftentimes use networks upon networks of actions that simulate complex decisions. Such a trait enables models to dynamically learn how to perform a specific task, and therefore can account for and properly respond to behavior that might have been otherwise unforeseen by a rule-based algorithm.
This concept can be applied to multiple areas of language learning. For simplicity, this article concerns a hypothetical “AI teacher” — that is, a personalized foreign language instructor constructed of machine learning technologies. Important parts of this instructor are brought to attention in separate sections. The first is as follows.
Phonetic Interfaces
The most common linguistic interface as of now is text. This could manifest itself as text conversations with a chatbot or sentences providing feedback on a certain assignment. Granted, when it comes to language learning, text is by no means the only means of communication. Especially when it comes to practical uses of language, speaking and listening can be equally important, but existing language education technologies have struggled to implement these aspects in a coherent, dynamic way. The reason is simple: it is extremely difficult to create a human-sounding voice on a computer, and companies often won’t take the risk of exposing budding students to a speech algorithm’s incorrect pronunciation or grammar. Moreover, due to the seemingly infinite number of tonal shifts and phonetic nuances within each language, it is quite difficult to create a standard algorithm that aptly encapsulates all the complexities of speech in the first place. It is, however, possible with AI.
Already, we’re seeing fluid AI generated speech in smart home devices such as Amazon Alexa, Google Home, or Siri. These technologies use a specialized form of AI (or “narrow AI”) that uses vast datasets of sample conversations, such as text queries and customer interactions, to learn to recognize and emulate human speech. No programmer is explicitly telling the algorithm how to speak like a human; the AI is learning itself based on statistical patterns observed from the data given. So provided there are sufficient amounts of sample data, these types of speech tools can be applied to almost any language. Admittedly, current models may very well still run into mistakes, but as larger datasets are compiled and more advanced models designed to capture the intricacies of natural speech are constructed, there is a very high likelihood that such interfaces would be able to seamlessly copy real speech.
The applications of this to language learning should be fairly straightforward. Currently, very few tools aid in improving pronunciation in a consistently high-quality manner. And even fewer technologies can hold full-on verbal conversations for the sake of improving conversational fluency. But as the frontiers of AI speech grow, both of these aspects will be sure to improve as well.
The Linguistic “Brain”
As of now, AI’s most popular task is solving problems. This could mean identifying cancer cells, predicting stock prices, or generating a recipe for oven-baked brownies. Regardless, recent developments in AI, specifically generative transformers (i.e., the G and T in GPT), have propelled models’ ability to understand queries and generate cohesive yet accurate responses. The key to this is a mechanism known as “attention” that is embedded within these transformers. Attention enables the algorithm to encode information about how one word in a sentence relates to every other word. In doing so, the model can get a better understanding of what the sentence could mean and how it could emulate that style.
This aspect of AI could, then, naturally suit the needs of grading, testing, and miscellaneous educational duties — all that would be needed is a prompt (or input query). To illustrate this, a couple examples are provided below:
- Cultural Conversation Bot: this prompt generates an interactive cultural conversation with the user as a tool to practice both language and culture in an authentic, practical setting.
- Language Conversation Bot: a more generalized version of the Cultural Conversation Bot provided above. The Language Conversation Bot can initiate a foreign language conversation in any specified setting, cultural or otherwise. Users can also specify learning level and other parameters by editing the prompt directly.
- Explain a Concept: explains a concept in detail. Any questions or unresolved issues can be addressed in follow up prompts (that is, prompts asked in response to the chatbot’s initial response). This is particularly useful for correcting misunderstandings of grammar or cultural concepts or ironing out topics with examples. That said, this can be used for any subject in any field — not just language learning.
Though the prompts require, first and foremost, user initiation, there are more implicit variations as well. For instance, if integrated properly, an AI-powered instructor could generate lesson plans or feedback without any explicit prompting. To illustrate the outcomes, here are some examples of how an AI would do this with prompting:
- Lesson Plan Designer: designs a lesson plan based on a topic, language, and learning level. Since most AI chatbots are trained on a wide range of online data, they are privy to current conventions and research on education and the psychology of learning new languages. As a result, all responses will be highly educated.
- Grammar or Spelling Error Detection: detects grammatical or spelling errors within a block of text. Note that there exist specific AI tools dedicated to such a field, such as the detection system for Grammarly. These centralized algorithms may be more effective than a generative AI alternative.
N.B. These are streamlined prompt tools that only take input fields and do not explicitly show the queries given to the chatbot. To access the queries, click the “Show Prompt” button and replace the placeholder phrases (the phrases in double curly brackets) with the input fields. These queries can be migrated to other generative AI chatbots. More information on all of these prompt tools can be found in other Lowtech articles.
What’s more is that this is all done through generative AI, which means that the model is trained to answer any question to a certain degree of accuracy. If a model were to be trained with the same resources to hone in on one of these tasks listed above, it might perform even better.
The Issue of Emotion
Although all these improvements are helpful, by themselves they’ll still suffer from an issue AI and other algorithms have struggled with since their creation — the lack of emotion. One of the main appeals of human teachers as opposed to AI ones is the connection between student and educator. The notion that AI can seem cold and heartless is by no means an insane or controversial one. If AI education truly wishes to make the next step, it needs to find a way to incorporate some sort of connection analogous to the one that can be found in the classroom.
Fortunately, there is work currently being done with respect to emotion and AI. This aspect is quite multifaceted, since it extends into both the generation of sentences, the linguistic “brain” as mentioned earlier, and potentially phonetic interfaces if those are implemented as well. Accordingly, the addition of emotion could entirely change the face of AI education and how it works with students.
A visual feature could even be added with the advent of emotion-related AI technologies. Having an AI instructor with a face or visual presence could strengthen a connection between instructor and student. Additionally, facial expressions enable emotion — and even personality — to be conveyed to a larger degree. The field of affective computing is already looking into the interpretation and expression of emotion, so perhaps in the near future, a convincing, fully-operational chatbot, fleshed out with emotion and personality, could come to fruition.
Conclusion
With the rapid pace of AI development, and how much every field is reaching out to incorporate some form of machine learning, it would not be surprising if researchers or companies implement most — if not all — of these features in the near future. And though the proposals above offer suggestions of ways in which AI can help language education, most likely someone will create a model or tool extremely useful to the field that would be unimaginable at the time of writing this article. That said, developers and researchers shouldn’t be blinded by the thought of progress. Language education and related fields have been long established and employ large swaths of the human population. Without proper care and regulation, a rapid shift to an AI-centered language learning environment could cause a sudden disruption to language teachers and the education system at large. And even though machine learning models and algorithms can emulate many different things, there are always aspects of human-to-human connection that will be irreplaceable. Whether it be actually immersing oneself in a different country or just speaking to a physical human being, human interactions can create a sense of engagement that an AI algorithm by itself simply can’t replicate.