A New Frontier in Financial Security Using AI

by Hagop Alajajian on Aug 7th, 2023
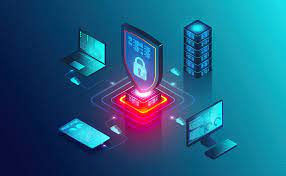
Security has been an issue for growing companies and professionals in finance for a long time now. And as the Internet continues to dominate the world, cyber security has become a more dominant issue for companies, especially financial firms, to deal with. In the context of financial security, generative AI has begun to be used for cyber security purposes, particularly through models such as generative adversarial networks. In the context of financial security, the application of this technology has transcended the conventional paradigms, introducing new methodologies to ensure integrity and safety. With the emergence of generative AI, there are various applications, techniques, and challenges associated with using AI for financial security.
Generative AI and Financial Forecasting
1. Fraud Detection
Utilizing generative models, financial institutions are now able to simulate fraudulent activities by creating synthetic data that mirrors real-world financial transactions. This, in combination with machine learning techniques such as deep learning and random forest algorithms, provides a multidimensional approach to fraud detection. The combination between generative AI and traditional machine learning augments real-time identification of fraudulent patterns, ensuring proactive measures.
2. Risk Management and Vulnerability Assessment
The application of Monte Carlo simulations, paired with generative models, enables the generation of a wide spectrum of potential outcomes, aiding in detailed risk assessment. By offering an insightful view of potential threats, these simulations provide an essential tool for financial analysts, allowing them to create more robust risk mitigation strategies.
Generative AI in Secure Data Handling
1. Data Anonymization
Data privacy, a cornerstone in financial services, can be significantly enhanced using generative models. By producing synthetic datasets that maintain the statistical integrity of original data, generative AI ensures compliance with stringent regulations like General Data Protection Regulation (GDPR). When businesses utilize synthetic data as a strategy for anonymization, they need to strike a median between utility and the degree of privacy safeguarding. Here, utility touches on the data’s analytical completeness and validity. The synthetic data must retain utility for analysis without jeopardizing individual privacy, thus enhancing both transparency and security.
2. Encrypted Transactions with Homomorphic Encryption
The combination of deep learning models with homomorphic encryption offers a novel approach to transaction processing. Homomorphic encryption involves changing text into a secret message using ciphertext, and so generative AI can be used to conduct the process of changing a message into ciphertext. By facilitating computations on encrypted data, these models ensure that sensitive information remains secure, even during the analytical process. This encryption method in combination with AI represents a leap forward in secure transaction handling.
Incorporating AI Techniques for Cybersecurity in Finance
1. Behavioral Analytics for Internal Threat Detection
The utilization of behavioral analytics algorithms, powered by AI, allows continuous monitoring of user activities within a financial system. By learning the normal behavioral patterns and promptly identifying deviations, these algorithms can preemptively detect potential internal threats.
2. Threat Intelligence through NLP and ML Models
The dynamic nature of cyber threats necessitates real-time intelligence. AI-driven systems employing natural language processing (NLP), including generative AI, and machine learning provide an efficient tool for scouring the internet and detecting emerging threats. This timely information empowers financial institutions to respond swiftly, reinforcing their security architecture.
3. Reinforcement Learning in Secure Communication Channels
Encryption algorithms can be optimized using reinforcement learning models, which learn and adapt to ever-evolving cyber threats. By ensuring the continuous evolution of encryption methodologies, these models play an integral role in maintaining secure communication channels.
Challenges and Ethical Considerations
The use of generative AI, although promising, raises complex challenges and ethical dilemmas. Ensuring the accuracy and integrity of synthetic data is paramount to prevent biases or errors that can lead to flawed decisions. Moreover, robust governance structures, coupled with ethical guidelines, must be implemented to prevent misuse of this technology for malicious purposes.
Future Uses
Generative AI introduces a sophisticated and nuanced approach to financial security, providing tools that enable fraud detection, risk management, and enhanced cybersecurity. To learn more about how to use AI to produce better cyber security for your company, use this prompt. Its integration with other AI techniques further fortifies the security architecture, promoting a more resilient financial ecosystem.
However, the pursuit of this innovative technology must be guided by caution, ethical principles, and an unwavering commitment to accuracy and integrity. The future of financial security could well be defined by the thoughtful and responsible implementation of generative AI, heralding a new era in which the financial system's stability and security are assured.